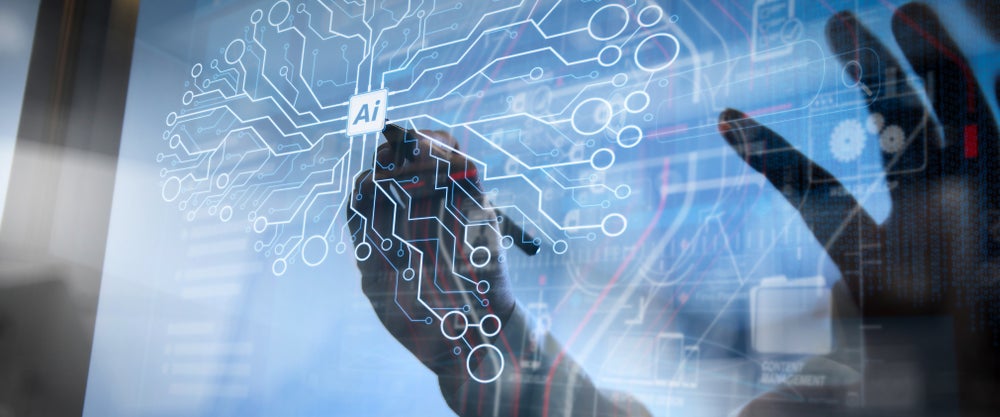
Artificial intelligence (AI) is set to be the most disruptive emerging technology in drug development in 2023, unlocking advanced analytics, enabling automation, and increasing speed across the clinical trial value chain.
Today’s clinical trials landscape is being shaped by macro trends that include the Covid-19 pandemic, geopolitical uncertainty, and climate pressures. Meanwhile, advancements in adaptive design, personalisation and novel treatments mean that clinical trials are more complex than ever. Sponsors seek greater agility and faster time to commercialisation while maintaining quality and safety in an evolving global market. Across every stage of clinical research, AI offers optimisation opportunities.
A new whitepaper from digital technology solutions provider Taimei examines the transformative impact of AI on the clinical trials of today and explores how it will shape the future.
Clinical trial patient recruitment and management
“The big delay areas are always patient recruitment, site start-up, querying, data review, and data cleaning,” explains Scott Clark, chief commercial officer at Taimei.
Patient recruitment is typically the most time-consuming stage of a clinical trial. Sponsors must find and identify a set of subjects, gather information, and use inclusion/exclusion criteria to filter and select participants. And high-quality patient recruitment is vital to a trial’s success.
Once patients are recruited, they must be managed effectively. Patient retention has a direct impact on the quality of the trial’s results, so their management is crucial. In today’s clinical trials, these patients can be distributed over more than a hundred sites and across multiple geographies, presenting huge data management challenges for sponsors.
AI can be leveraged across patient recruitment and management to boost efficiency, quality, and retention. Algorithms can gather subject information and screen and filter potential participants. They can analyse data sources such as medical records and even social media content to detect subgroups and geographies that may be relevant to the trial. AI can also alert medical staff and patients to clinical trial opportunities.
The result? Faster, more efficient patient recruitment, with the ability to reach more diverse populations and more relevant participants, as well as increase quality and retention. “[Using AI], you can develop the correct cohort,” explains Clark. “It’s about accuracy, efficiency, and safety.”
Shortening study build timelines for clinical trials
Study build can be a laborious and repetitive process. Typically, data managers must read the study protocol and generate as many as 50-60 case report forms (CRFs). Each trial has different CRF requirements. CRF design and database building can take weeks and has a direct impact on the quality and accuracy of the clinical trial.
Enter AI. Automated text reading can parse, categorise, and stratify corpora of words to automatically generate eCRFs and the data capture matrix. “In study building, AI is able to read the protocols and pull the best CRF forms for the best outcomes,” adds Clark.
It can then use the data points from the CRFs to build the study base, creating the whole database in a matter of minutes rather than weeks. The database is structured for export to the biostatistician’s programming. AI can then facilitate the analysis of data and develop all of the required tables, listings and figures (TLFs). It can even come to a conclusion on the outcomes, pending review.
Optical character recognition (OCR) can address structured and unstructured native documents. Using built-in edit checks, AI can reduce the timeframe for study build from ten weeks to just one, freeing up data managers’ time. “We are able to do up to 168% more edit checks than are done currently in the human manual process,” says Clark. AI can also automate remote monitoring to identify outliers and suggest the best route of action, to be taken with approval from the project manager.
AI data management is flexible, agile, and robust. Using electronic data capture (EDC) removes the need to manage paper-based documentation. This is essential for modern clinical trials, which can present huge amounts of unstructured data thanks to the rise of advances such as decentralisation, wearables, telemedicine, and self-reporting.
“Once the trial is launched, you can use AI to do automatic querying and medical coding,” says Clark. When there’s a piece of data that doesn’t make sense or is not coded, AI can flag it and provide suggestions automatically. “The data manager just reviews what it’s corrected,” adds Clark. “That’s a big time-saver.” By leveraging AI throughout data input, sponsors also cut out the lengthy process of data cleaning at the end of a trial.
AI in clinical research: from idea to implementation
Implementing AI means establishing the proof of concept, building a customised knowledge base, and training the model to solve the problem on a large scale. Algorithms must be trained on large amounts of data to remove bias and ensure accuracy. Today, APIs enable best-in-class advances to be integrated into clinical trial applications.
By taking repetitive tasks away from human personnel, AI accelerates the time to market for life-saving drugs and frees up man-hours for more specialist tasks. By analysing past and present trial data, AI can be used to inform future research, with machine learning able to suggest better study design. In the long term, AI has the potential to shift the focus away from trial implementation and towards drug discovery, enabling improved treatments for patients who need them.
To find out more, download the whitepaper below.