
The need for new medical treatments and drugs has never been greater. But before pharmaceutical companies can go to market with a breakthrough drug, they need to ensure safety and efficacy through clinical trials. While this process is essential, it’s also slow, expensive and unpredictable. Pharma R&D teams are solving this problem by leveraging the power of artificial intelligence (AI) in clinical trials to save time and money.
Drug development is already a difficult endeavor, with the vast majority of R&D efforts failing to produce a market-worthy product. Even reaching the clinical trial phase offers no guarantees, as only 12% of such drugs receive U.S. Food and Drug Administration approval. Pharma companies need tools like AI that can reliably improve this percentage without jeopardizing safety.
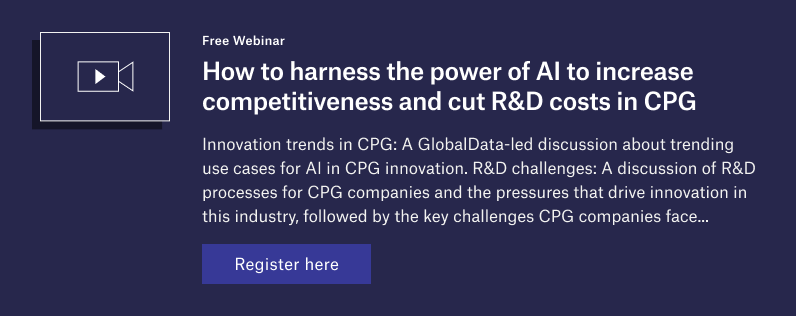
Learn more about the benefits and challenges of AI and clinical trials, as well as examples of AI’s use in this industry.
Overview of the drug development process
Drug development is a complex and costly process that typically takes years to complete. Before a new medication can be approved for use, it needs to go through rigorous testing and evaluation in clinical trials. These trials involve screening voluntary participants and monitoring their responses. AI and machine learning are increasingly leveraged to accelerate drug development and optimize clinical trial outcomes.
The process of drug development typically starts with the identification of a potential target for a drug. The target could be an enzyme or receptor in the human body, or it could be an organism that causes disease. Once the target is identified, researchers work to develop a compound that can interact with it. After a promising compound has been identified, it’s tested in preclinical models to evaluate its safety and efficacy. Only then does it progress to clinical trials with human participants.
Clinical trials are divided into phases evaluating drug safety, side effects and effectiveness from increasingly larger pools of candidates and patients. During these phases, data is obtained from participants and monitored for adverse reactions or changes to health indicators, such as blood pressure or heart rate.
AI and clinical trials
AI-based technologies are increasingly being used in preclinical studies and clinical trials. This helps companies improve the accuracy and efficiency of testing, accelerate drug development and optimize outcomes. Advanced algorithms, for example, can quickly analyze vast databases of chemical compounds and identify those most likely to bind to a target. Algorithms can also predict the toxicity of a compound and its potential side effects, allowing researchers to focus their efforts on the most promising candidates.
AI also helps in interactions with patients, as it can be used for automating patient recruitment and data collection. AI-based analysis can provide insight into participant behavior that informs how researchers design trials.
Cutting-edge pharma companies are also using AI to detect patterns within the vast amounts of data generated by clinical trials. These patterns might reveal trends that would be difficult or impossible to detect using traditional methods. AI can analyze this data in real time, allowing researchers to quickly identify trends and make informed decisions about how to proceed with trials. The result of all these improvements is better drug discovery, better-designed and safer clinical trials, and improved patient outcomes at lower costs than ever.
One of the biggest advantages of leveraging AI is the ability to find insights and expand current knowledge beyond human capabilities. As clinical trials generate a massive amount of data, it can be challenging for researchers to manually review all of this data to uncover meaningful insights. For example, important information may be locked in knowledge silos or gained through experience, making it difficult to find or document.
To solve this problem, companies are also using the power of AI to provide greater access to knowledge, which helps improve collaboration, efficiency and performance. Starmind, for example, uses machine learning algorithms to bring additional value to your team and the insights discovered by AI. The platform allows employees to easily connect with subject matter experts that can share past experiences and provide support for a specific topic. This can lead to faster problem-solving, better decision-making and, ultimately, more successful clinical trials.
6 benefits of leveraging AI in clinical trials
Companies know about the power of AI in pharma, but realizing that potential is more difficult. It takes time to understand AI’s applications and to figure out how to deploy it most effectively in their workflows. Here are key advantages of leveraging AI in clinical trials.
Faster time to market
One of the biggest benefits of AI is its ability to automate many of the time-consuming and labor-intensive tasks associated with clinical trials. These include data collection and analysis, and cohort and patient selection. For example, AI algorithms can analyze vast amounts of patient data to identify high-potential candidates for a clinical trial. Algorithms also can analyze patient behavior patterns and make recommendations for trial design.
Tools powered by AI, such as Starmind, can also streamline R&D time to market by improving access to knowledge and enabling more effective collaboration within the organization. When R&D teams can easily share and access key information across the enterprise, such as recruitment data or trial results, they improve communication and collaboration while avoiding duplicate work.
Reduced costs
AI reduces the costs associated with clinical trials by streamlining the drug development process and making it more efficient. By automating workflows such as patient recruitment and safety monitoring, R&D teams save time and money. Predictive analytics tools, meanwhile, help companies make better decisions about resource allocation.
AI can also speed up crucial steps in the pharma R&D process, such as the discovery process for novel drug compounds or the design of clinical drug trials.
More accurate data analysis
Researchers can use algorithms to analyze large amounts of data quickly and identify patterns and trends that clinical researchers would overlook or take much longer to spot.
For example, AI-based models can be used to predict the toxicity of potential drug candidates, allowing research teams to rule out compounds that aren’t suitable for further development. Safety is the most prominent benefit here, but these AI models also save companies time and money on efforts that are unlikely to yield results.
Personalized medicine
Every patient has unique needs and challenges, which adds to the difficulty in testing treatment effectiveness. AI can help identify specific patient populations that are most likely to benefit from a particular drug based on factors such as genetic makeup and lifestyle. With the help of AI, personalized medicine can become a reality.
Other ways AI can personalize treatment include dosing, where AI tools can determine the right dosage amount and frequency based on the patient’s characteristics, including personal and family medical history.
Improved patient outcomes
AI contributes throughout the R&D process to treatments that are more effective and have fewer side effects. This benefit begins in the drug discovery process by ruling out unpromising approaches, and it continues through to clinical trial design, patient recruitment and safety monitoring.
Every discovery, whether of a new drug or a new application for an existing drug, increases the options for patients in need. AI is a powerful tool for this purpose, and not just for new drugs. Using AI to analyze existing drugs and treatments is an effective way to uncover new applications. After all, AI is well-suited for identifying patterns in patient data and drug characteristics.
Ahead of clinical trials, AI can improve efforts to recruit high-potential candidates or predict which patients are at a higher risk of experiencing adverse reactions. This improves the quality of your patient pool and trial design.
The patient experience during clinical trials can also be improved with AI. Decentralized clinical trials, for example, enable remote monitoring and data collection. Wearable devices and mobile apps collect real-time data, such as vital signs and medication adherence, and transmit it to researchers. Algorithms can analyze patient data and provide early warning signals, allowing for quicker intervention. Meanwhile, AI-based reinforcement learning methods can improve participant adherence to trial protocols.
Real-time access to expertise
The complex nature of the pharmaceutical industry makes it vital for teams to have access to up-to-date information and research. It’s also important to have a central location for teams to find and share knowledge based on previous research and experiences, and to connect with subject matter experts that can bridge any knowledge gaps.
Many organizations also suffer from information silos between teams or departments. Too often, the answers a team seeks have already been found by colleagues, but that knowledge isn’t accessible or visible.
AI can break down these silos by building a comprehensive knowledge network and connecting teams with subject matter experts for additional information. Centralizing organizational knowledge makes collaboration easier, speeds up innovation and helps teams get answers quickly and return to work. With the right knowledge solution, not only are experts accessible, their knowledge can be automatically documented and stored for future use.
Improved access to knowledge has a direct impact on overall performance. More than two-thirds of R&D companies say projects have been delayed due to missing information. In pharma, these delays can put patients’ health in jeopardy while resulting in millions of dollars in lost revenue.
5 examples of AI for clinical trials
By using AI-driven algorithms for a wide range of tasks, from data entry and analysis to improved medical diagnoses, companies can reduce the time and cost associated with drug development. Here are some ways companies are using AI in clinical trials.
Patient recruitment and screening
AI technologies help clinical researchers do a better job of patient recruitment and screening. Algorithms can sift through large amounts of patient data to quickly identify potential participants based on specific inclusion and exclusion criteria.
AI-powered chatbots and virtual assistants can provide patients with information about the trial, answer their questions and collect preliminary data. This reduces time and resources spent on manual patient screening and interviewing.
Trial design and optimization
By finding patterns in data, AI improves trial design and optimization through predictions about patient behavior and drug efficacy. With this added knowledge, researchers can design more efficient and effective clinical trials while identifying the most suitable patient populations, treatment regimens and dosages.
For example, researchers could use Starmind to determine the optimal patient population for a trial by analyzing data from previous studies and identifying which patient populations responded best to a particular treatment. They can also use the tool to identify potential challenges or risks, avoid duplicate work and consult with experts to optimize the trial and improve the likelihood
Data collection and analysis
One of the main benefits of AI is its ability to automatically collect and analyze data. In pharma R&D, this can include data from electronic health records, administrative records and health surveys. This allows for more comprehensive and accurate data collection and management, which in turn can lead to more robust trial results.
Predictive modeling
Using predictive modeling, researchers can identify patient populations best suited for specific treatments and adjust trial design accordingly. This improves the chances of success and reduces the risk of trial failure or patient harm. Predictive modeling also identifies potential safety concerns earlier in the drug development process.
Adverse event detection
Adverse events, or unexpected side effects, are a crucial aspect of clinical trials. Traditional methods of adverse event detection rely on manual reporting by participants and healthcare professionals, which can be time-consuming and error-prone.
AI streamlines this process by identifying potential adverse events more quickly and accurately than traditional methods. By using machine learning algorithms to analyze data from multiple sources, including electronic health records, patient-reported outcomes and social media, AI identifies potential adverse events early on. The benefits include fewer serious incidents, saved time and better trial results.
Natural language processing
Natural language processing (NLP) is a subfield of AI that focuses on the interaction between computers and human languages. In clinical trials, NLP can be used to extract and analyze unstructured data from various sources, such as electronic medical records and patient-reported outcomes. NLP automates data extraction and analysis, which saves time and resources. It can also help researchers find patterns and relationships within the data that might otherwise be missed or take too long to determine otherwise.
The future of AI in clinical trials
AI in clinical trials is already a reality, and its use will only grow throughout the drug development process. Researchers have more data available than ever before and more powerful tools to analyze it. But what many researchers are missing is real-time access to global experts and past examples to optimize their drug development process.
With Starmind’s AI solution, companies can effectively use AI to centralize knowledge, unlock sharing and connect employees with subject matter experts within the organization.
The world’s leading biotech companies trust Starmind to help their team members connect to expert knowledge all over the world as they work towards doubling their medical advances at half the cost to society.