In 2021 and throughout Q1 2022, the pharmaceutical sector has continued to forge strategic partnerships with artificial intelligence (AI) vendors. Merck’s latest bet is to generate clinical evidence—suitable for supporting regulatory decisions—using digitally simulated ‘predicted outcomes’ rather than actual patients. The pharmaceutical company has signed a multi-year collaboration with Unlearn.AI, a start-up that promises to slash the time and costs of clinical research while maintaining trial power and confidence, by enrolling ‘Digital Twins,’ virtual replicas of existing patients.Â
The first Digital Twin was developed by NASA to mirror the Apollo 13, informing engineers in Houston of the predicted health and trajectory of a rocket launched into outer space. Using biomedical terms, a Digital Twin could be defined as ‘an experimental model recapitulating the complexity of a system which, in response to an event, behaves in a statistically indistinguishable way from its real counterpart’.
How well do you really know your competitors?
Access the most comprehensive Company Profiles on the market, powered by GlobalData. Save hours of research. Gain competitive edge.
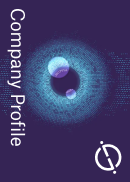
Thank you!
Your download email will arrive shortly
Not ready to buy yet? Download a free sample
We are confident about the unique quality of our Company Profiles. However, we want you to make the most beneficial decision for your business, so we offer a free sample that you can download by submitting the below form
By GlobalDataUnlearn.AI’s Digital Twin technology relies on two proprietary pipelines. The first, PROCOVA, is a machine learning method in which historical datasets are used to identify prognostic markers and prognostic scores and produce a generative model to predict the clinical evolution of a disease. The second, Twintelligent RCTs, is an AI-powered algorithm assessing baseline clinical records of a specific patient against the generative model to forecast a comprehensive, longitudinal clinical record that describes what would have happened if that specific patient had received a placebo. This approach reduces by 30% the number of patients needed for the study. Some real control patients are still required for a final algorithm to perform quality control that, in the absence of bias, should not be able to distinguish the real from the simulated dataset.
In comparison to existing synthetic control arms (SCA), this approach controls bias by avoiding the introduction of external comparators. In theory, this approach is superior even to gold-standard randomised controlled trials, as it represents a univariate analysis in which the only difference between the patients and their twins is in the treatment received. Moreover, PROCOVA offers a unique advantage: the generative model does not try to rationalise the survival of patients simply by using their clinical profile. Instead, it allows for the existence of known and unknown confounding variables that will always result in a mismatch between a diagnostic forecast and reality. By modelling uncertainty, then, the AI elegantly controls this bias and corrects it without needing to fully characterise human complexity. The ability to control bias throughout the pipeline has been applauded by the US Food and Drug Administration (FDA), which was actively involved by the developers.
On 22 March 2022, the European Medicines Agency (EMA) also released a preliminary approval for PROCOVA, allowing the use of this technology in Phase II and III trials in the European Union.
See Also:
On 19 April, Unlearn.AI closed a $50m Series B funding to advance the use of Twintelligent RCTs in clinical trials. The company has built databases that cover central nervous system (CNS) disorders—the AI vendor’s training ground—and several therapeutic applications reflecting Merck’s pipeline products. Unlearn’s CEO’s mention of a second phase of the collaboration might point towards a vision of exporting Digital Twins across the therapeutic applications covered. So, what are the implications for oncology?
The FDA currently considers SCA to be the best scientific evidence when it is complicated or not ethical to have a control arm, such as in paediatric settings. A formalisation of the FDA guidelines on AI in clinical trials would likely see AI replacing external control groups.
A wider application of Digital Twins in late-phase trials, however, appears more complicated. In oncology, investigational new drugs are compared with standard treatments, so each twin would act both as a prognostic and a predictive forecast. Different generative models would be required for each therapeutic regimen, classification, and position, among others. Because of its complexity, cancer would require at least three -omics datasets (likely, copy number variation, methylation status and RNA-seq), and the dynamic evolution of the disease would quickly invalidate the model and require a new one. Two specific qualities of this machine learning method could, however, potentially play an important role in oncology other than its primary designated use.
First, for every new patient, the model continues to learn about the disease; and second, the way the Bayesian network generates predictions makes PROCOVA ideal for taking an event that occurred and predicting the likelihood that any one of several possible causes was the contributing factor. At present, 1,700 clinical trials are trying to identify patients likely to respond to the PD-1 inhibitor Keytruda across a wide variety of treatment settings. The use of AI and machine learning can offer powerful tools to answer these questions, as well as enable smaller, more efficient trials that maintain power and result in faster and cheaper therapies for patients.
Related Company Profiles
Merck & Co Inc