Need to Know:
- AI can identify suitable cohorts for clinical trials by analysing medical records and social media content.
- Trial recruitment can be sped up by using AI technologies to alert medical staff and patients about trial opportunities, as well as simplifying entry criteria to be more accessible for potential participants.
- While AI has shown potential benefits, universal data management and reduction of bias in data is needed to employ AI efficiently.
The initial stages of population selection and patient recruitment can influence the success of a clinical trial.
While patient selection and recruitment can be a time- and resource-consuming process, a successful determination of participants will increase trial efficacy potential, explains Stefan Harrer, PhD, chief innovation officer at Digital Health CRC. However, unsuccessful cohort determination and recruitment are amongst the worst monetary burdens, he adds.
If trial recruitment is not successful and quick, it can lead to a trial failure. This potential financial loss should be considered an incentive to use artificial intelligence (AI) technologies in the initial stages of clinical trials, says Harrer.
AI technology can examine through large amounts of data to detect patient subgroups that might benefit more in a clinical trial. It can also analyse social media content to identify specific regions where a condition is more prevalent, thus narrowing down the search for the right cohort.
AI has the potential to speed up the process of finding eligible participants by analysing hospital medical records and alerting both clinicians and patients about clinical trial opportunities. The technology can also simplify complex entry criteria and make it more presentable to potential candidates.
How well do you really know your competitors?
Access the most comprehensive Company Profiles on the market, powered by GlobalData. Save hours of research. Gain competitive edge.
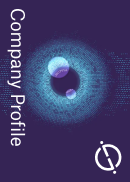
Thank you!
Your download email will arrive shortly
Not ready to buy yet? Download a free sample
We are confident about the unique quality of our Company Profiles. However, we want you to make the most beneficial decision for your business, so we offer a free sample that you can download by submitting the below form
By GlobalDataHowever, while AI is a promising tool to make clinical trial recruitment stages more efficient, it still faces many unknowns. Data interoperability and inherit bias in currently available data are the issues that need to be addressed to deploy the use of AI successfully and efficiently in clinical trials.
Identifying and selecting cohorts
AI technology can help sift through large amounts of medical health records and generate data which can help to find eligible populations for a clinical trial.
For example, researchers at Mount Sinai Medical Center in New York used a topological data analysis (TDA) to identify three subgroups in type 2 diabetes by analyzing electronic health records and genotype data. The TDA provided patterns of clinical characteristics and disease comorbidities, which provided insights on how specific patients would respond to a medication or in a clinical trial. However, Harrer notes that the final decision regarding patients should be made by a human, while AI should be seen as an additional layer in the process.
“The combination of assistive power of AI and the existing experience of clinicians can maximise the chances of making the best possible decision and minimize the chances of losing information,” he adds.
One of the most advanced parts of utilising AI in patient identification is through analyzing social media content. AI can be used to mine though online forums where patients exchange information about their conditions to detect if there are certain locations or regions where a medical condition might be more prevalent. This approach could help clinical trial organizers to speed up cohort identification, which would help companies design clinical trials effectively, says Harrer.
OPYL has developed a technique that helps identify patient populations by looking at publicly generated content on networks like Reddit, Twitter or Quora, says Michelle Gallaher, CEO of OPYL. Social media content can be valuable when trying to learn about patient experience regarding their condition, she adds. Recently, the company helped a neurological study in distress to identify patients with Alzheimer’s disease after receiving interest from potential study populations that did not have a clear diagnosis. By using predictive technology and analysis of digital biomarkers in the cohort’s behaviour on social media, OPYL identified people with a diagnosis of Alzheimer’s disease and predicted their geographical location, explains Gallaher.
Patient recruitment
Once a population is selected, AI can help with recruitment. Traditionally, eligible patients are found though hospitals or clinics, but often when recruiting large numbers of people, only a few will be suitable for a trial, explains Dr. Arun Bhatt, an India-based clinical research and drug development consultant. AI technology can be used to optimize the recruitment process and reduce the amount of unnecessary screening.
Alastair Denniston, PhD, director of INSIGHT, a health data research hub for eye health, explains that there is an opportunity to use a simple, rules-based AI approach to recruit patients. For example, when provided with eligibility criteria, an automated system can generate a list of potential participants by examining a hospital database. This list can be used by clinicians to inform their patients about their eligibility or the patients themselves can be contacted directly by the system. However, the latter needs to be handled carefully with the supervision of a clinician to ensure people are not pressured to participate, says Denniston.
Data mining helps to find eligible, informed, and motivated patients, says Harrer. A technique called Natural Language Processing (NLP) can analyse written medical records and the eligibility criteria of clinical trials that are publicly available. As the terminology used in trial criteria descriptions may be too complex for a patient to understand their eligibility, NLP can translate it into layman’s terms and make it more accessible to prospective patients.
Challenges in using AI
While there are some cases showing the benefits of AI in population selection and recruitment, the integration of AI technology into clinical trials faces challenges. Medical record data comes in different formats and levels of quality, making it hard to effectively employ AI in generating new sets of data. Having a unified global approach to collecting and using data would be ideal, but as every country has different regulations, it will be hard to combine all information into one format, says Bhatt.
Interoperability and access to data are the key ingredients to developing an AI algorithm, but the current data challenges makes it hard to come up with a coherent pathway to develop this technology, says Harrer. One of the most important tasks in AI development is creating data management platforms. This will improve the technology itself and empower digital health solutions.
Another issue that AI is facing is the inherit bias in currently available data. Denniston explains that genetic databases are predominantly focused on patients of European descent. When such datasets are used to train an algorithm, it can leave out significant parts of the population that are historically not represented.
Bias can also be seen in endpoint selection, such as the perception of pain in a certain condition, says Gallaher. The perception and management of pain from a patient’s perspective is completely different than clinical records or published literature. There is a need to find a way to combine these two sets of data to have a two-sided view of pain in a condition.
“For too long, we’ve ignored the patient-reported experiences and not given patients the opportunity to create data and share that experience, particularly not in clinical trials,” Gallaher says, adding that patients should be seen as partners in research rather than participants.
Internal versus external AI collaborations
Introducing AI companies as a third party into a clinical trial can cause ethical issues for patients who do not want to share their confidential information with a tech company, thus driving them away from a clinical trial, says Bhatt.
To combat that, pharma companies should build internal divisions that are solely focused on data and AI solutions, says Harrer. This has been a noticable trend in mid- and large-size companies. “Data science, digital and especially AI analytic capabilities are recognised as key tools in the core pharma toolbox,” he adds.
However, outsourcing a company that works with AI can bring valuable relationships with AI developers in academia, explains Gallaher. She explains that some of the values of an external service provider are the constant search for new technologies, and deep networks with academic AI developers, which grants quick access to new technologies.
Due to the large size of some pharma companies, it can be it hard for them to navigate relationships with academic institutions that develop AI technology. The bigger the company is, the longer it takes establish these relationships, which does not correlate with the fast development of AI processes.
Moreover, a service provider can offer a healthy barrier between the pharma company and the patient. Most pharma companies do not want to handle generated patient experience data or have a direct contact with them, as they prefer insights on the data alone, said Gallaher.