Data Integrity has been on the agenda of regulatory authorities since the introduction of 21 CFR Part 11 in 19971. Before that the need to address data integrity issues was illustrated by ‘the generics scandal’ in the 1980s where falsified data was submitted to support drug approvals2.
A common myth is the statement that data integrity is a new requirement of cGMP. In 2003 ‘Part 11, Electronic Records; Electronic Signatures — Scope and Application’ was published followed by an announcement in 2010 of the FDA, stating that the agency will be conducting inspections focusing on 21 CFR Part 11 requirements3. This announcement has been followed accordingly with data integrity now being a primary inspection focus and with the presence of highly qualified inspectors trained specifically in data integrity.
Today, the regulatory literature has been expanded significantly since the emergence of 21 CFR part 11 giving further guidance in authorities’ interpretation in cGMP requirements and their own guidelines. A list of relevant available literature is provided below:
- US FDA 21 CFR Part 11
- EudraLex, Volume 4 Good Manufacturing Practice (Annex 11)
- MHRA GMP Data Integrity Definitions and Guidance for Industry March 2015
- FDA Guidance for Industry Data Integrity and Compliance with cGMP April 2016 (draft)
- WHO Guidance on Good Data and Record Management Practices September 2015 (draft)
Spectrum of Data Integrity Issues
When studying warning letters given by regulatory authorities it is evident that the term ‘data integrity’ covers a wide range of issues. These issues can vary in degree of severity in a spectrum as shown in Figure 1:
Figure 1 – Spectrum of data integrity issues pending from minor bad practices to full-blown falsification scandals
How well do you really know your competitors?
Access the most comprehensive Company Profiles on the market, powered by GlobalData. Save hours of research. Gain competitive edge.
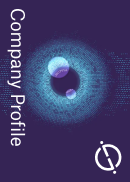
Thank you!
Your download email will arrive shortly
Not ready to buy yet? Download a free sample
We are confident about the unique quality of our Company Profiles. However, we want you to make the most beneficial decision for your business, so we offer a free sample that you can download by submitting the below form
By GlobalDataExamples of actual warning letters containing these categories are given below:
Table 1 – Examples of data integrity issues referencing actual warning letters
Working with Data Integrity in Practice – ALK’s process for auditing data
The objective of performing an audit of data during development is to provide an assessment of the data quality based on a statistically sound data audit sampling plan, as well as a data audit sampling strategy. The amount of source documentation and data generated prior to regulatory approvals are extensive and the data generated has (hopefully!) already been verified prior to the reporting of results. Therefore, the most meaningful way of auditing the data is by developing a solid, statistically sound sampling plan. The scope of ALK’s data auditing process is summarized in Figure 2:
Figure 2 – Scope of Audit of Data Review performed by QA
The verification and execution of audit is performed by QA and with involvement of relevant areas where source documentation and data have been generated. Furthermore, a sampling plan has been designed taking into consideration parameters such as:
- AQL (Acceptable Quality Level): defined as the level of document quality (percent defective) the sampling plan routinely accepts
- RQL (Reject Quality Level): defined as the level of document quality (percent defective) the sampling routinely rejects
- Accept number (a): Defective count that, if exceeded, will subject the verified document to appropriate corrective actions
- Lot size of population (N): in this context the total number of data points in a document
- Sample size (n): Total number of data points sampled from a document
The set values that are to be used for AQL, RQL, a, N, and n must be determined by the individual company. Our experience at ALK has shown that it is mandatory to include a skilled statistician in these considerations. Furthermore, we have implemented error categories, such as Critical, Major and Minor for detected investigational findings (data errors), while also establishing gradual AQL and RQL levels for the different error categories based on criticality.
Data Integrity is for the patients
Ultimately, data integrity compliance is for the sake of the patients. Data integrity compliance and thereby trustworthy, accurate and complete data is necessary for assuring medicines’ and medical devices’ safety, efficacy and quality. The challenges faced when determining which statistical parameters and parameter levels to be used are challenging as well as exciting.
Â
Â
References:
1. Guidance for Industry Part 11, Electronic Records; Electronic Signatures — Scope and Application – http://www.fda.gov/downloads/RegulatoryInformation/Guidances/ucm125125.pdf
2. Joseph P. Reid, Generic Drug Price Scandal: Too Bitter a Pill for the Drug Price Competition and Patent Term Restoration Act to Swallow, 75 Notre Dame L. Rev. 309 (1999).
3. FDA link (FDA To Conduct Inspections Focusing on 21 CFR 11 (Part 11) requirements relating to human drugs) –http://www.fda.gov/AboutFDA/CentersOffices/OfficeofMedicalProductsandTobacco/CDER/ucm204012.htm