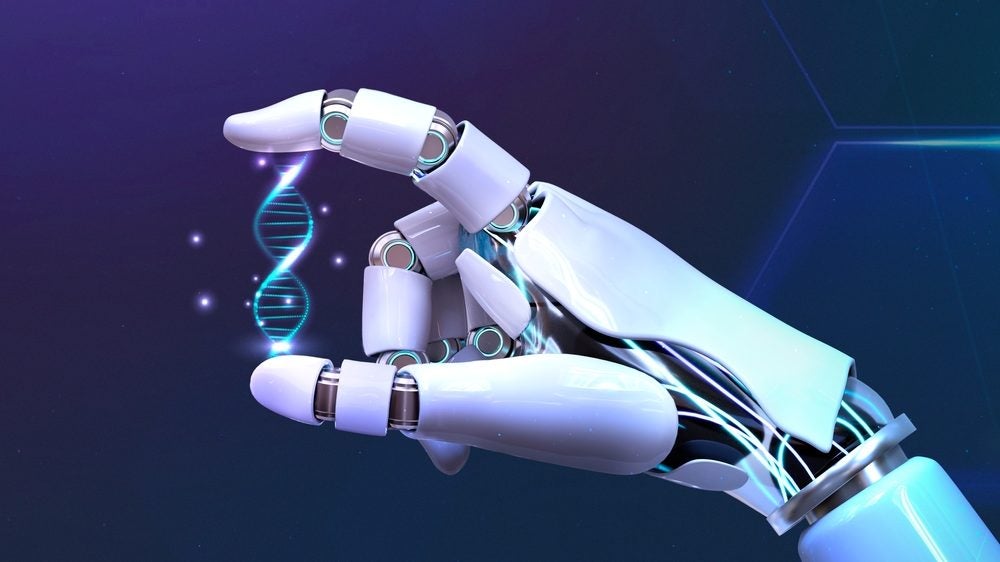
Even if artificial intelligence (AI) seemed like a sci-fi technology just a few years ago, it has become obvious that AI is here to stay as tools like ChatGPT and generative AI have been making headlines in the past few months. Certainly, AI has asserted itself in the biotech industry.
Biotech companies have been exploring how they can utilise AI and machine learning (ML) in drug discovery and development. AI tools like BioGPT have shown human parity in answering biomedical research questions and some companies are starting trials with their AI-generated drugs.
Yet, there are some challenges in sight for AI and ML to become a solid reality. With such fast-paced innovation, the desired talent pool and regulatory decisions are lagging. To this end, a panel of experts sat together for a roundtable discussion on 24 October in London, which was hosted by biotechnology cloud-based platform provider Benchling, to discuss the current state of play of AI and biotech.
Employing younger professionals
One of the main challenges in the successful AI/ML adoption is the availability of skilled talent, according to Benchling’s “2023 State of Tech in Biopharma Report”, which was published in September. Emmanouil Metzakopian, PhD, vice president of research and development at bit.bio, explains that even though AI has been around for a while, recently there has been an offshoot of technological updates. This resulted in restrictive sets of experts who are knowledgeable on the use of new technologies.
Additionally, there is an inherited tendency to hire people with extensive scholarly degrees and knowledge, says Pierre Salvy, PhD, head of engineering at biotech Cambrium. While experience is certainly needed, young people with bachelor’s degrees are excited to utilise their academic knowledge. “People are underestimating how smart younger students are,” he adds.
See Also:
A lack of talent pool could also be seen as a cultural gap within companies. Often, there is a disconnect between departments, especially when it comes to employees from the computational and R&D sectors. At Cambrium, machine learning engineers start their onboarding process by learning how painstaking it can be to generate data to avoid resentment later, explains Salvy. “Data collaboration between the people are foundational solutions to these challenges,” he adds.
How well do you really know your competitors?
Access the most comprehensive Company Profiles on the market, powered by GlobalData. Save hours of research. Gain competitive edge.
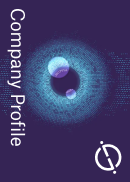
Thank you!
Your download email will arrive shortly
Not ready to buy yet? Download a free sample
We are confident about the unique quality of our Company Profiles. However, we want you to make the most beneficial decision for your business, so we offer a free sample that you can download by submitting the below form
By GlobalDataSo, how does one learn the different languages that are spoken between departments? Uzma Choudry, PhD, tech and biotech investor at Octopus Ventures, says that this needs to be worked on early on in the education system so there would be more cross-pollination to avoid siloed disciplines. “When you do not have as much exposure to the other language, the bridging of those gaps becomes much harder,” she notes.
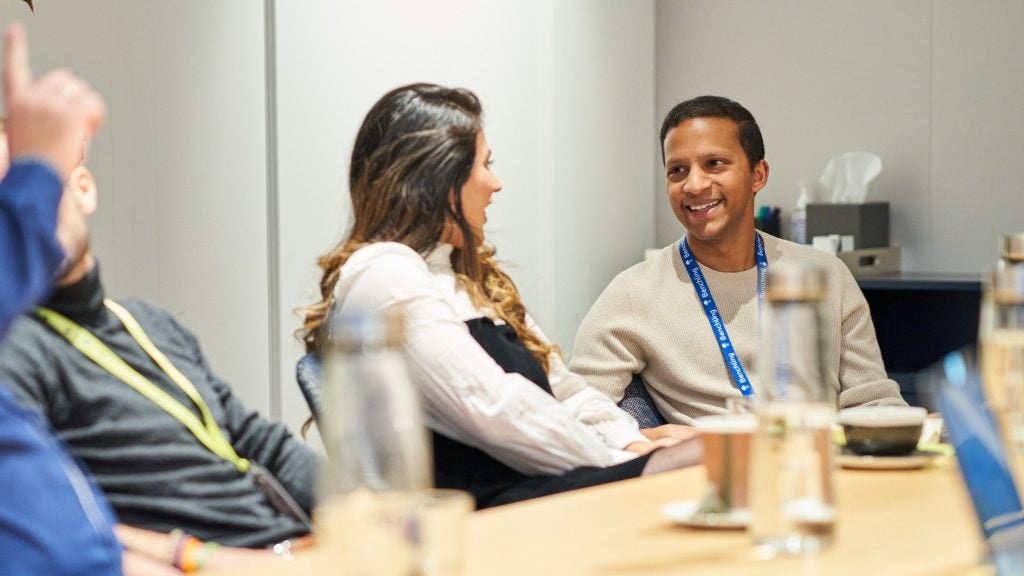
Such cultural challenges are especially tough on organisations that have been operating for a while and found their success without AI-driven technologies, explains Benchling’s CEO Saji Wickramasekara. On the opposite, digitally native start-ups can be built from the ground up where leadership has a mix of data science, ML and biology. However, the recent popularity of ChatGPT has piqued the interest of scientists who are now more eager to use AI tools, he adds.
Is there enough regulation?
As AI continues to advance, regulatory agencies across the globe are trying to catch up with it. Earlier this year, both the US Food and Drug Administration (FDA) and European Medicines Agency (EMA) released discussion and draft reflection papers to spur the conversation around AI/ML in drug development and the lifecycle of medicines.
However, the question arises: should technology drive regulations or should regulations influence technological advancements? Metzakopian says that because everything is moving so fast, the industry needs to discuss the progress with the regulators and work with them closely. Salvy also notes that there needs to be friction for discussions to go forward, and the industry should ask itself which regulations are the right ones to be passed.
On the other hand, James Field, PhD, CEO at biotech LabGenius, says that some people lean into the idea of regulations because it gives a sense of control. “The reality is that it is all moving so fast and we have to be mindful around applying additional regulation,” he explains, adding that therapeutics are already regulated sufficiently.
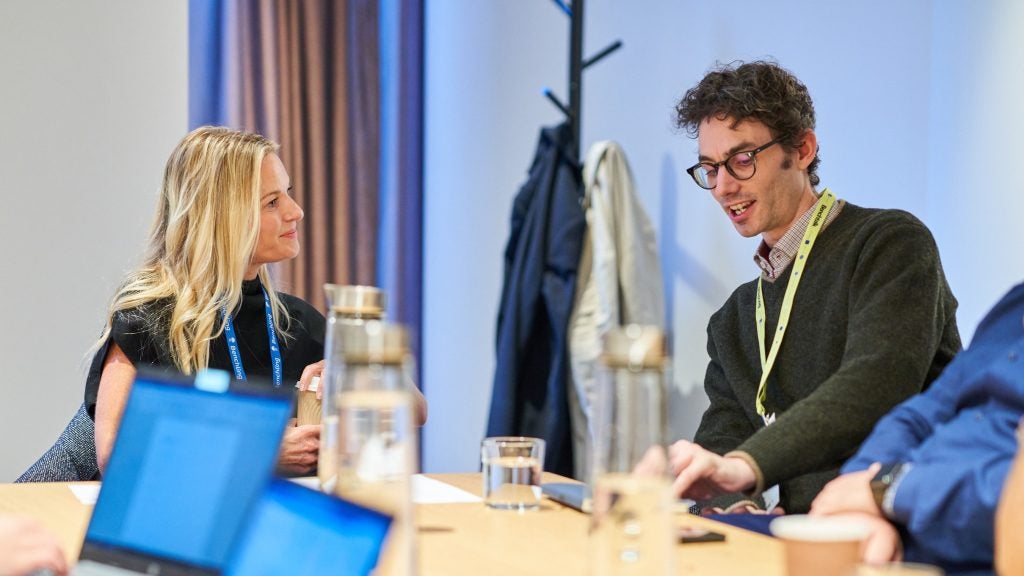
In any case, AI-powered drug development needs to apply the same safety and risk assessments as any research would do, Metzakopian explains. Such risk assessments will also pinpoint who is liable for the decision-making, as questions were raised about safety and liability. In addition, Salvy says that predictions that are generated by machine models are the responsibility of the operator who is running it. He adds that it is good to call attention to the liability issue as there is a need to educate the general public about this complicated topic.
Measuring the impact and success of AI
Respondents to Benchling’s report ranked AI/ML the lowest in terms of potential impact compared to other tech tools such as R&D data platforms, robotics and automation, and connected instruments and equipment.
This low rating boils down to oversimplification of AI and ML. However, AI/ML is imbued at every single step in the scientific processes, Field explains. Salvy adds that AI/ML also has a big impact on human health as it is bringing patients closer to personalised medicine since AI can predict the outcome of the molecule. While personalised medicine is currently costly, over time it should become inexpensive for the masses, says Wickramasekara. He notes that medicine is one of the few fields that costs less as time goes by compared to other fields.
Choudry says that improving patient outcomes and experiences are a few ways that investors use to measure the success of AI. On the drug discovery side, success is measured by the clinical asset pipeline as ML allows companies to explore new chemical and biological spaces. Also, increasingly, investors are looking at what data companies have on their asset pipelines to evaluate the company, she adds.
What is certain is that AI will speed up better therapeutic development, especially in unmet need indications or therapy areas. Still, Field notes that a lot of work needs to be done as some of the first AI-developed therapeutics have failed in trials. “The reality is that we will not know if this works until the end,” he adds.