On January 26, Microsoft announced that the artificial intelligence (AI) tool BioGPT demonstrated “human parity” in analyzing biomedical research to answer questions. But like many recent advances in AI, evaluating what the new technology actually means for healthcare can prove challenging.
BioGPT is a type of generative language model, trained on millions of previously published biomedical research articles. This means BioGPT can perform tasks such as answering questions, extracting relevant data, and generating text relevant to biomedical literature.
For example, as a potential drug development application, BioGPT can generate descriptions of a specific therapeutic class—such as “Janus kinase 3 (JAK-3)”—or of a specific therapy—such as “Apricitabine.” (In a demo version of BioGPT, users can test the text generation feature in a limited capacity).
Still, to fully grasp the implications of BioGPT, it is important to understand what researchers know and do not know about the potential breakthrough AI technology.
How does BioGPT work?
BioGPT relies on deep learning, where artificial neural networks—meant to mimic neurons in the human brain—learn to process increasingly complex data on their own. As a result, the new AI program is a type of “black box” technology, meaning developers do not know how individual components of neural networks work together to create the output.
How well do you really know your competitors?
Access the most comprehensive Company Profiles on the market, powered by GlobalData. Save hours of research. Gain competitive edge.
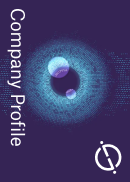
Thank you!
Your download email will arrive shortly
Not ready to buy yet? Download a free sample
We are confident about the unique quality of our Company Profiles. However, we want you to make the most beneficial decision for your business, so we offer a free sample that you can download by submitting the below form
By GlobalDataTo assess the accuracy of generative AI models, researchers have developed tests to measure natural language processing (NLP)—or the ability to understand text and spoken language. Microsoft’s recent paper assessed BioGPT along six scales of NLP, reporting that the new model outperformed previous models on most tasks. This includes the well-established scale PubMedQA, in which Microsoft reported BioGPT achieved human parity.
In PubMedQA, users must answer “yes,” “no,” or “maybe” to a series of biomedical questions based on corresponding abstracts from the database PubMed. For example, one PubMedQA prompt asks, “Do preoperative statins reduce atrial fibrillation after coronary artery bypass grafting?”
BioGPT-Large, the most extensive version of the AI program, achieved a record 81% accuracy on PubMedQA, compared to an accuracy of 78% for a single human annotator. Most other NLP programs, including Google’s BERT family of language models, have not surpassed human accuracy.
What are the limitations of BioGPT?
BioGPT works similarly to ChatGPT, the software from Open AI that made waves with its release last December. Though BioGPT is trained specifically on biomedical literature, it still carries many of the same limitations as ChatGPT—and AI more broadly.
Still, there are growing concerns that generative language models can produce inaccurate text without any references, potentially disseminating misinformation. In addition, BioGPT trains on existing medical research that could carry biases, causing the AI program to risk perpetuating these same biases.
The rise of BioGPT forms part of the wider push towards AI solutions in healthcare and the clinical trials industry. Recently, AI has shown the potential to improving clinical trial patient selection, predicting drug development outcomes, and developing digital biomarkers.