Scientific and technological advancements continue to shake the conservative nature of the pharmaceutical industry. Newer technologies like artificial intelligence (AI) have the potential to improve patient identification, adherence and retention, and the development of digital biomarkers. Today, complementary approaches that have been around for some time, such as predictive analytics, continue to solidify and expand their uses in drug development and clinical trial design.
The concept of predictive analytics is fairly simple – it is the ability to use data, algorithms, and visualisations to forecast a certain outcome or event that may or may not happen in the future. While predictive analytics and AI have overlapping areas, the former is more hypothesis-driven and statistical methods are needed to test that hypothesis, whereas AI is hypothesis-free, meaning the machine itself is asking questions about the data.
Even though AI might sound more advanced and effective, its black-box nature needs to be balanced out by the stability of predictive analytics. Clinical Trials Arena talks to experts about drug development and clinical trial design areas that can be powered by predictive analytics and the role of AI. Also, they discuss the current regulatory uncertainty and what needs to be done to improve the fair and safe use of algorithms.
Potential replacement of animal studies
Last September, the US Senate passed the FDA Modernisation Act 2.0, which authorised the use of alternatives to animal testing, such as cell-based assays and computer models. While this change has been sought by animal welfare organisations, animal testing was not the most accurate method to predict how an investigational drug will work in humans, says Dr Eric Perakslis, chief science and digital officer at the Duke Clinical Research Institute.
For example, a tumour which was grown in a dish and injected under the skin of a mouse does not represent how a real tumour grows in a person. “It’s a foreign body in the mouse so the immunological response is going to be different,” he explains, adding that computational models might get closer to a real representation of the biological activity in a human.
However, because predictive analytics only predict the outcome, this method will need the help of AI to build digital animal models, says Dr Michael Pencina, vice dean for data science and director of Duke AI Health at Duke University School of Medicine. “This could be a replacement for some of the animal testing. The science is so advanced that we can make a lot of progress,” he adds. As previously reported in Clinical Trials Arena, computational models might accelerate the development of inclusive drugs for the pregnant population.
How well do you really know your competitors?
Access the most comprehensive Company Profiles on the market, powered by GlobalData. Save hours of research. Gain competitive edge.
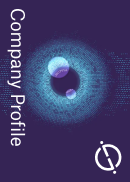
Thank you!
Your download email will arrive shortly
Not ready to buy yet? Download a free sample
We are confident about the unique quality of our Company Profiles. However, we want you to make the most beneficial decision for your business, so we offer a free sample that you can download by submitting the below form
By GlobalDataAs with every methodology, there are certain limitations as to how accurate these predictive models are. “If you create perfect digital mice, will the technology be sufficiently representative and able to account for the modifications to the mice or the treatment and predict what nature is going to do or evolve to?” questions Pencina.
Dr Jens Fiehler, director of the neuroradiology department at the Hamburg University Hospital and managing director at Eppdata GmbH, is doubtful that predictive analytics will fully replace animal studies. “To simulate a biological environment with all the hormones and enzymes is quite difficult and complex,” he notes.
A realistic mindset is needed. Pencina explains that there are real-life cases where AI or predictive analytics have failed “spectacularly”. For example, a paper published in 2019 identified racial bias in predictive analytics algorithms of people who need extra healthcare resources. While it was the fault of the experiment design and not the algorithm, it shows that human insights are needed to avoid potential damage, which would eventually affect human perception. “One accident by a self-driving Tesla carries much more concern in public perception than the thousands of accidents caused by humans,” Pencina notes.
However, Perakslis argues that there is more to come, and improvements in analytical capabilities will further show the potential of predictive modelling. “Just because it failed in the last 40 years, doesn’t mean it’s going to fail in the next 20 years,” he says.
Sample size calculation based on historical data
While the replacement of animal studies is still in question, predictive analytics have shown promise in other areas of clinical trial design. A recently published paper looked at borrowing historical data and using predictive analytics to calculate a sample size for a trial. Sample size calculation is one of the lower bar applications of predictive analytics, as such calculations are very much driven by assumptions, Pencina says.
Yet, this use case is interesting only to a point, Perakslis notes. He explains that regulatory authorities might be suspicious of trying to lower the costs and increase the speed of a trial. “If a traditional power calculation says 500 patients and your analytics say you can do it in 158, you will probably not sell that to a regulator,” he adds.
A bigger and more promising avenue for predictive analytics is borrowing historical controls, Pencina says. For example, if a trial is designed to do a one-to-one randomisation and 1,000 people are recruited to the placebo or standard of care (SOC) arms, the sponsor can enrol 500 actual patients and borrow the other 500 from historical data.
Predicting outcomes of a simulated intervention
Predictive analytic models can also act as virtual comparators. Fiehler conducted a thrombectomy study investigating if a next-generation device improves outcomes in patients with ischemic stroke while simulating an outcome of an alternative treatment.
Such an approach is very beneficial in the endovascular procedure field as most of the trials are single-arm studies which are using literature-based comparators. Randomised control trials are also not feasible as they are too expensive, need twice as many patients, and it takes such a long time that the device might not be in the market anymore, Fiehler explains.
The study used pre-treatment and post-treatment image datasets to train a machine-learning model. Then, the pre-treatment data was used to model the infarct size if a patient received medical therapy. By the end, researchers had a simulated outcome for the medical therapy and an observed outcome from the thrombectomy trial.
While this approach allows two outcomes in one individual to be compared, the integrity of training datasets is crucial. Fiehler explains that while it is not hard to get hold of such data, it must be credible and used in a very transparent and audited way.
The wild west of algorithms
Even though predictive analytics have been around for a long time, authorities are still catching up on how to regulate their use in the clinical environment. Pencina calls it “a wild west of algorithms” as people are developing and selling algorithms with very low accountability and a limited amount of testing. While well-defined guardrails are needed, he is cautious about heavy-handed regulations which may stifle innovation.
Because it is already expensive to develop these algorithms, the only people who will be able to play in a highly regulated space are the big pharma companies. “You don’t want to create a world where your regulation is well-intentioned but too heavy-handed that you give a competitive advantage only for the big ones to become bigger,” Pencina explains.
Perakslis suggests that the FDA needs to start a new realm of regulatory science for algorithms and predictive analytics. In the same way the FDA started the Center for Biologics Evaluation and Research (CBER) to regulate medical products without “a discrete ingredient list”, the agency needs to use that logic for algorithms, as a lot of AI-powered technologies generate unreadable code to a human. In the absence of expertise and regulations from the FDA, the agency recommends sponsors or developers to seek outside input for external validation, especially if the software product gets into more serious use, he adds.
In the next few years, studies using predictive modelling will be done in parallel, says Fiehler. Using predictive analytics as secondary endpoints or additional analysis will gain more trust and better understanding from people. “It is not witchcraft or rocket science,” he says. “But we need to work together on a framework, where to integrate the models, and what are the standards for the data that are used to train the models.”
Takeaways:
- AI-powered predictive analytics might replace aspects of animal testing. However, it is still unclear how accurate these predictive models will be.
- Predictive analytics can be used for sample size calculation and as a virtual comparator in certain medical fields. Data integrity and transparent methods are needed to improve the adoption.
- Regulators are still catching up on how to regulate predictive analytics and its algorithms. Yet highly regulated frameworks might stifle innovation.